Time Series Analysis is a vast field in itself, where we analyze data points in an equally spaced sequence of discrete-time data.
For example, by closely looking at weekly Sales patterns for a Brand at a Store, to Inventory positions in a time series can reveal insights where we can analyze seasonality, trends, cyclical and even noise to gain meaningful statistics and other data related insights.
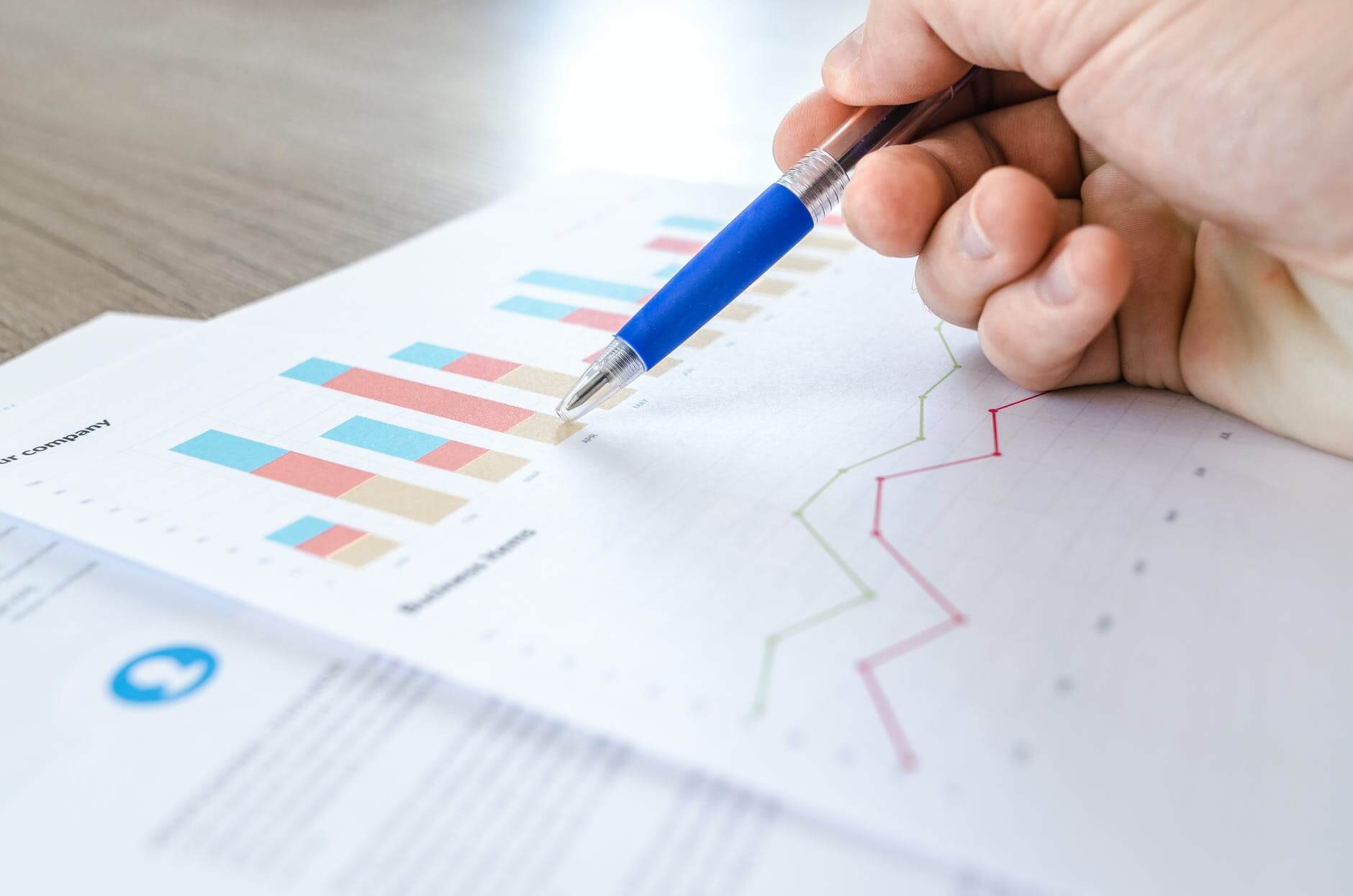
Using the Descriptive and Diagnostic pillars of analytics, a time series can reveal WHAT IS GOING ON and perform a causal analysis on WHY something happened. In the above example, time series analysis can reveal why Sales dipped for a week vs. the previous week.
In this post, let me throw some focus on using Index numbers to measure Price, Units, etc., in a time series – where the Index reflects the % differences between the Base period (Year, Month, Week, Day, etc.) and the selected period.
Using Index numbers has been one of my favorite way of comparing and presenting data over time. It provides a uniform base (Index of 100), and we can easily compare the percentage increase or decrease vs. the Base. For example, if last year’s Sales Index was 100 and this year it increases to 105, it means a 5% increase in Sales. Similarly, this year’s Index of 95 means Sales has dipped 5% compared to last year.
Have a look at the image below to get a closer look at Indexes.
Using the formula as stated above, we can arrive at the Sales Index of 106.8 by doing the math- (135379312/126733283)*100
You can try it next time by plotting the calculated Indexes in a time series – it will surely make the comparisons easier to understand and more impactful!
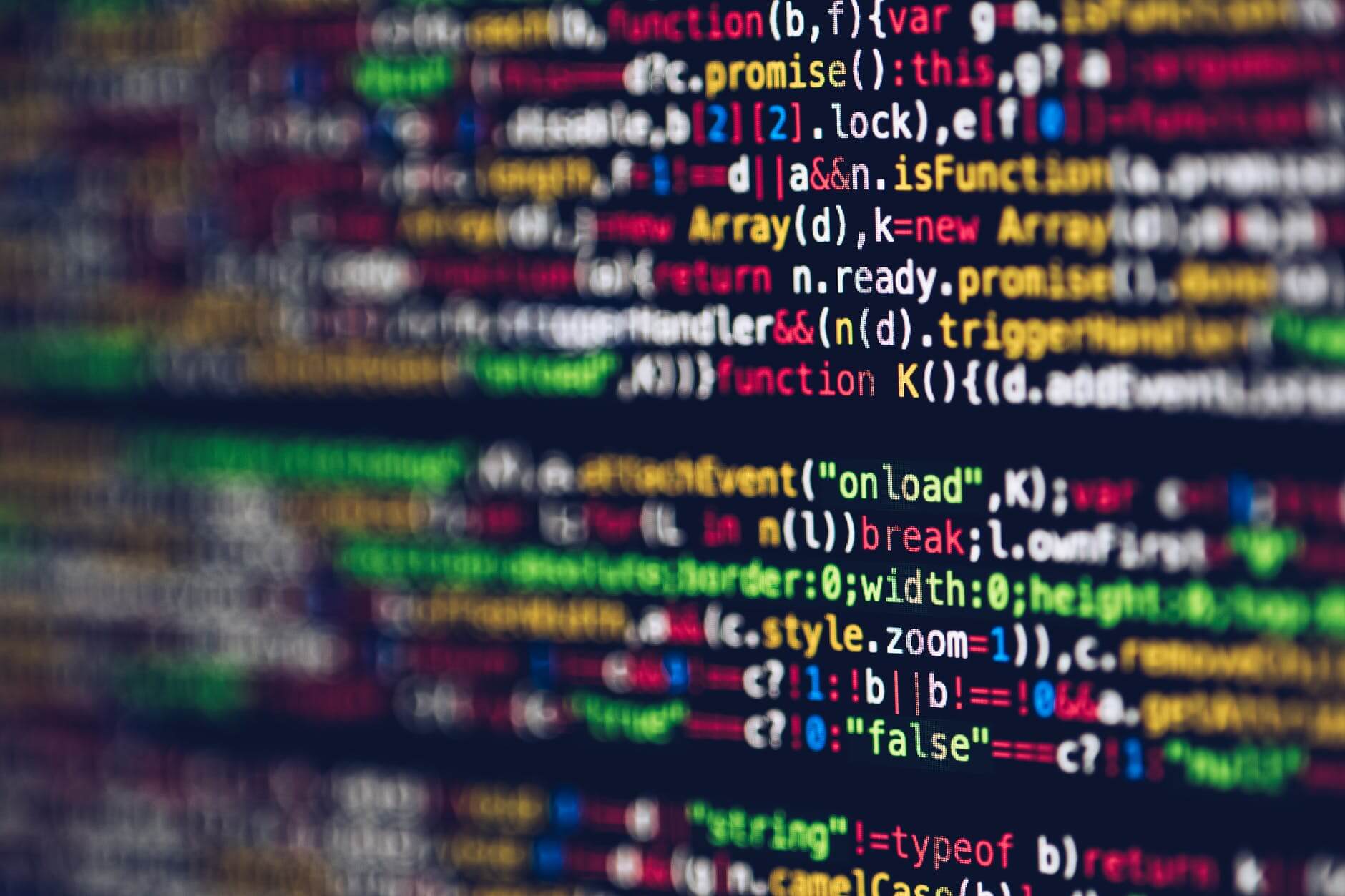
Adios!
Comments are closed.